Qian Tao
Time and again, AI-methods such as deep learning have proven their value in medical imaging for clinical diagnosis and treatment guidance. But sometimes they fail for no obvious reason, and they tend to be overconfident. After more than a decade of AI research at LUMC, rising star Qian Tao now focusses on improving trust in AI from a more technical perspective.
‘I decided to study electrical engineering because that is what the cool kids do at the time,’ Qian Tao says. ‘And a girl doing that is especially cool!’ Optional courses in medical imaging settled the future course of her career. ‘Using physics to probe inside the human body, and then do mathematical analysis to make sense of it. It is simply fascinating.’
With AI, we can analyse Gigabytes of cardiac MRI data in less than a second
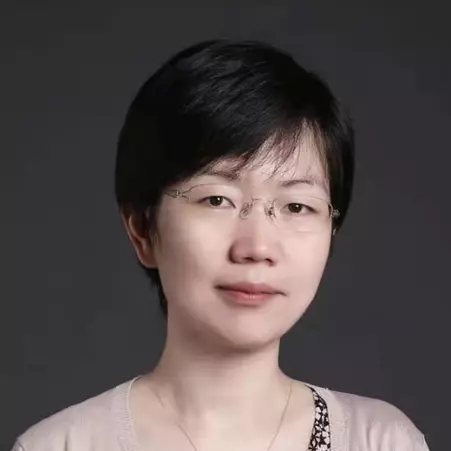
Cardiac MRI
After her PhD she joined the Division of Image Processing, Department of Radiology of the Leiden University Medical Center, focussing on cardiac MRI – an advanced technique for heart imaging. ‘With MRI, you can assess the beating heart with a high temporal and spatial resolution. You can non-invasively reveal scarring and fibrosis on the heart muscles, which are the underlying cause of many cardiac diseases. Using AI methods we can extract such features from Gigabytes of MR image data in less than a second.’
Her AI-methods guide electrophysiologists when performing catheter ablation, reducing the time of the procedure and X-ray exposure to both the patient and the physician. The extracted features also serve as input for classical risk stratification models of cardiac mortality. Tao: ‘We are working on new methods, using AI for direct risk stratification.’
A rigorous step forward
Although really enjoying the close collaboration with radiologists and cardiologists, she decided to adjust her course and join TU Delft as an assistant professor in the Department of Imaging Physics. ‘I always have clinical applications in mind, and still maintain many active collaborations with clinical experts, but I wanted to pursue a more technical focus.’ she says. ‘To bring AI to the next stage, we need to study the rigour of these algorithms. And adding a reliable measure of uncertainty to AI output, for example, can increase the trust of the end-users, such as radiologists and cardiologists. I would also like to “see” inside AI – to understand its inner workings. TU Delft provides a wonderful platform for me to do all this research.’
For one, she is now co-director of the Chemical Engineering & Medical Imaging AI Lab (CHEME AI Lab), strengthening data-driven AI by integrating fundamental scientific knowledge. ‘It adds prior knowledge and boundary conditions, such that the output of an AI algorithm is properly regularized, increasing the fitness of the outcome even with insufficient or unexpected data.’
Adding a reliable measure for uncertainty to AI output can increase the trust of the end-users, such as radiologists and cardiologists
New Adventure
Qian was very excited to join the Department of Imaging Physics at TU Delft because of the abundant complementary expertise of her physicist colleagues. ‘They create the most advanced images using physics, high-dimensional and high volume, for which AI can play an important role in the analysis,’ she says. ‘I’d love to explore more possibilities, as the high-level principles of AI for image analysis, in the end, are the same.’
Having only recently joined TU Delft, she is exploring collaborations within TU Delft, with Erasmus MC and LUMC, and outside the Netherlands. ‘I was awarded a DHI seed grant together with a colleague from Erasmus MC,’ she says.
Mystery buster
In her time off, Qian enjoys travelling with her family. ‘I hope my children can experience and explore all the nice and different cultures. And Europe is a wonderful place to travel.’ At work, she will continue to do her own exploring. ‘Deep learning still has many mysteries, and it is the perfect time to work on them. I do believe they can be resolved, and I wish to be a part of it, especially for medical imaging applications.’
Deep learning still has many mysteries