BIOLab
Biomedical Intervention Optimisation lab
Modern machine learning algorithms have achieved unprecedented accuracy in image and video understanding tasks, via pure learning from data. These powerful abilities come at the price of enormous amounts of training data, memory, and computational requirements. Such resources are rarely available to real-time feedback systems in medical intervention and biomedical research.
Experts will join forces in the BIOLab across many fields including computer vision, reinforcement learning, neural architecture, deep learning and computational physics, and biomedical imaging. We will create high-efficiency, real-time, AI-driven feedback and control in biomedical applications. The focus is on improving the efficiency of machine learning algorithms by designing novel artificial neural network architectures, developing new reinforcement learning and generative algorithms, and incorporating biologically inspired neural network models. These newly developed concepts and algorithms will be applied to a wide range of problems in biomedical applications. Examples include optimizing tumour irradiation protocols with missing information, and limiting irradiation damage to delicate living samples in smart microscopy.
The BIOLab is part of the TU Delft AI Labs programme.
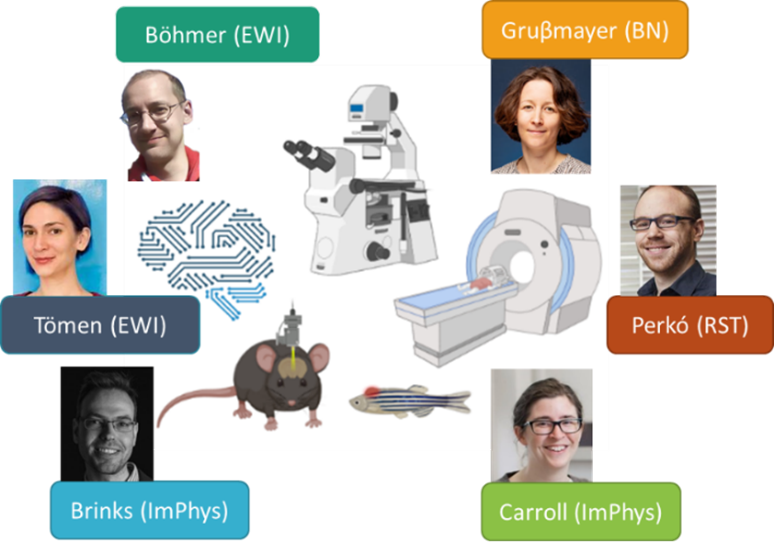
The Team
Directors
PhD's
Education
Courses
2022/2023
- Advanced Optical Imaging | AP3122
- Medical Physics of Proton and Photon Therapy | AP3582
- Interdisciplinary Advanced Artifial Intelligence Project | IFEEMCS520200
- Data Mining | CSE2525
2021/2022
- Capstone Applied AI Project | TI3150TU
- Fundamentals of AI | IFEEMCS520100
- Image analysis | NB2121
2019/2020
- Machine Learning 2 | CS4230
- Deep Learning | CS4240
- Image Analysis | NB2121
- Seminar Computer Vision by Deep Learning | CS4245
- Research Project | CSE3000
- Final Project | IN5000
- AI in Computational Neuroscience | NB2041
- Research Practicum | TN2953P
Master projects
Ongoing
- Smart Super-resolution Microscopy Data Acquisition Using Deep Learning, Nergis Tomen, Gijs Schout (2023/2024)
- A biophysical model for enhanced understanding of learning processes in the inferior olive, Daan Brinks, Jaume Abad i Villa (2023/2024)
- On- and off-target effects of non-normal perturbation dynamics in small and large recurrent cortical networks, Daan Brinks, Niek Rijnders (2023/2024)
- Machine-learning assisted Directed evolution for protein engineering, Daan Brinks, Rowan Brakel (2023/2024)
- Modelling of Physically accurate neural networks for applications in reinforcement learning, Daan Brinks, Ian van Vliet (2023/2024)
- Time steps in spiking neural networks, Nergis Tomen, Alex de Los Santos Subirats (2023/2024)
- Self-organized criticality in spiking networks of non-leaky integrator neurons, Nergis Tomen, Luca Frattini (2023/2024)
- NeuroBench: Benchmarking spiking and analog neural networks for primate tracking sata, Nergis Tomen, Paul Hueber (2022/2023)
- Making It Clear Using Vision Transformers in Multi-View Stereo on Specular and Transparent Materials, Nergis Tomen, Pieter Tolsma (2022/2023)
Finished
- Evaluation of the influence of shape priors in deep learning based cardiac segmentation, Nergis Tomen, Denzil Joy (2022/2023)
- Simple Online Visual Object Tracker Fusion based on Distributed Kalman Filtering, Nergis Tomen, Yigen Zhong (2022/2023)
- Optimizing Event-Based Vision by Realizing Super-Resolution in Event-Space: an Experimental Approach, Nergis Tomen, Mahir Sabanoglu (2022/2023)
- Optical Flow Upsamplers Ignore Details: Neighborhood Attention Transformers for Convex Upsampling, Nergis Tomen, Sander Gielisse (2022/2023)